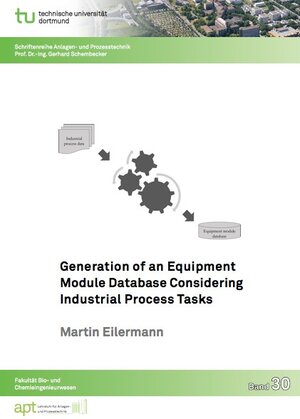
×
Generation of an Equipment Module Database Considering Industrial Process Tasks
von Martin EilermannModule-based plant design opens up the opportunity for the (bio-)chemical industry to reduce lead times, which is crucial for future competitiveness. Suitable equipment modules are selected from an Equipment Module Database instead of time-consuming tailor-made equipment design. Thereby, engineering work can be reused. Although of central importance in module-based plant design, an applicable Equipment Module Database does not yet exist.
Therefore, within this work, two approaches for the generation of an Equipment Module Database are developed and compared. Both approaches are based on process tasks with industrial relevance in order to generate equipment modules competitive with conventional equipment design.
One approach is based on the idea that similar process tasks can be performed by the same equipment module. Characteristic features are a measure for the similarity of process tasks. Within this work, an approach to determine these characteristic features is presented. Based on these features, existing industrial process tasks are grouped by hierarchical cluster analysis. For each cluster of process tasks a representative equipment design is selected from a set of equipment module candidates. These candidates are generated quasi-randomly based on a Sobol’ sequence. The representative equipment designs are stored in the Equipment Module Database.
The other approach is independent of clusters of process tasks and considers the generation of an Equipment Module Database as a maximum coverage problem known from other disciplines. Within this work, the corresponding maximum coverage problem is formulated and solved by a randomized greedy algorithm.
Both approaches for the generation of an Equipment Module Database are successfully applied to existing industrial heat exchanger and condenser tasks provided by Evonik. It is shown, that the approach based on the maximum coverage problem leads to better results. Nevertheless, the characteristic features determined can still be applied during equipment module selection as presented within this work.
Therefore, within this work, two approaches for the generation of an Equipment Module Database are developed and compared. Both approaches are based on process tasks with industrial relevance in order to generate equipment modules competitive with conventional equipment design.
One approach is based on the idea that similar process tasks can be performed by the same equipment module. Characteristic features are a measure for the similarity of process tasks. Within this work, an approach to determine these characteristic features is presented. Based on these features, existing industrial process tasks are grouped by hierarchical cluster analysis. For each cluster of process tasks a representative equipment design is selected from a set of equipment module candidates. These candidates are generated quasi-randomly based on a Sobol’ sequence. The representative equipment designs are stored in the Equipment Module Database.
The other approach is independent of clusters of process tasks and considers the generation of an Equipment Module Database as a maximum coverage problem known from other disciplines. Within this work, the corresponding maximum coverage problem is formulated and solved by a randomized greedy algorithm.
Both approaches for the generation of an Equipment Module Database are successfully applied to existing industrial heat exchanger and condenser tasks provided by Evonik. It is shown, that the approach based on the maximum coverage problem leads to better results. Nevertheless, the characteristic features determined can still be applied during equipment module selection as presented within this work.