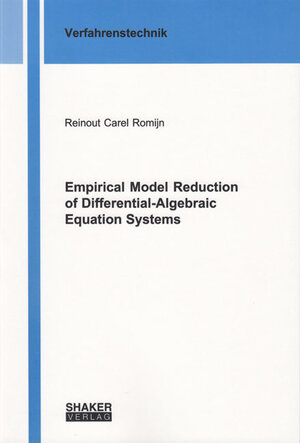
×
Empirical Model Reduction of Differential-Algebraic Equation Systems
von Reinout Carel RomijnThis thesis addresses the reduction of differential-algebraic equation (DAE) systems. Modelling of physical or chemical processes often results in an equation system with both differential and algebraic equations. For various applications (e. g. applications in real-time) the simulation time of the model should be sufficiently short, which might not be the case when the model size is too large. In order to reduce the simulation time, mathematical methods for model reduction are deployed. In this thesis, a new method based on proper orthogonal decomposition (POD) is proposed for the reduction of linear and nonlinear DAE systems which performs better than the POD methods available in literature.
The proposed POD method involves a system transformation which separates the DAE system into a dynamic subsystem and an algebraic subsystem before reducing the system by a Galerkin Projection. The connection between the POD reduction method and the balanced truncation reduction method which exists for ODE systems is generalized to DAE systems in this way. Linear DAE systems with an arbitrary differential index can be reduced by the proposed method in contrast to the POD methods previously proposed in literature that might fail for systems with a differential index larger than one. The various empirical reduction methods are also applied to a nonlinear DAE system with strangeness index zero. The newly proposed method is able to reduce this type of system, where one of the classical methods fails.
Since reducing the size of a nonlinear system by projection does not always result in a shorter simulation time, a grey-box modelling approach is proposed, which replaces the high number of computationally expensive nonlinear functions by a smaller number of parametrized nonlinear functions.
The proposed POD method involves a system transformation which separates the DAE system into a dynamic subsystem and an algebraic subsystem before reducing the system by a Galerkin Projection. The connection between the POD reduction method and the balanced truncation reduction method which exists for ODE systems is generalized to DAE systems in this way. Linear DAE systems with an arbitrary differential index can be reduced by the proposed method in contrast to the POD methods previously proposed in literature that might fail for systems with a differential index larger than one. The various empirical reduction methods are also applied to a nonlinear DAE system with strangeness index zero. The newly proposed method is able to reduce this type of system, where one of the classical methods fails.
Since reducing the size of a nonlinear system by projection does not always result in a shorter simulation time, a grey-box modelling approach is proposed, which replaces the high number of computationally expensive nonlinear functions by a smaller number of parametrized nonlinear functions.