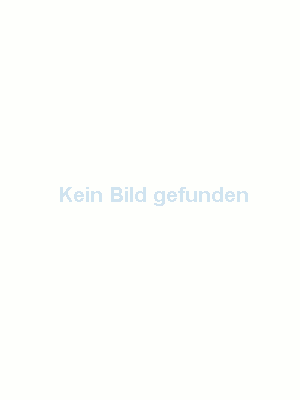
Asymptotic Expansion and Weak Approximation
Applications of Malliavin Calculus and Deep Learning
von Akihiko Takahashi und Toshihiro YamadaThis book provides a self-contained lecture on a Malliavin calculus approach to asymptotic expansion and weak approximation of stochastic differential equations (SDEs) as well as numerical methods for computing parabolic partial differential equations (PDEs). Particularly, Malliavin’s integration by parts is effectively applied to the computation schemes combined with deep learning methods. Constructions of asymptotic expansion and weak approximation are given in detail with the theoretical convergence analysis. The schemes enable efficient computation for high-dimensional SDEs and fast spatial approximation for high-dimensional parabolic PDEs without suffering from the curse of dimensionality. Moreover, the algorithms and Python codes are available with numerical examples for finance, physics, and statistics. Readers including graduate-level students, researchers, and practitioners can understand both theoretical and applied aspects of recent developments of asymptotic expansion and weak approximation.