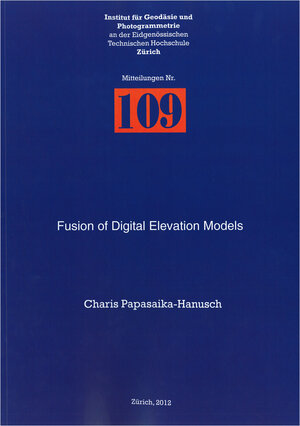
×
Fusion of Digital Elevation Models
von Charis Papasaika-HanuschDigital Elevations Models are one of the most important types of geodata. They are needed in a large number of applications, ranging from virtual globes and visualization to engineering and environmental planning. DEMs of larger areas are usually generated either by photogrammetric processing of aerial and satellite images, InSAR interferometry, or laser scanning (mainly from airborne platforms). Each sensing technology has its own strengths and weaknesses, and even within one technology the variations in DEM quality are large. DEMs are available at different scales from tailor-made local models to national and even global coverage. We are primarily interested in large-scale national and global products, whose resolution, accuracy, error characteristics, and homogeneity vary a lot. In most cases, the DEM producers provide users with information only on production technology, date of acquisition, and resolution, but only with coarse accuracy measures that fail to capture the local variations in data quality – sometimes only a single global number.
In an ideal world, one would of course obtain the raw measurements and sensor models from all sensors, and merge them by fitting a single DEM to the entire set of heterogeneous observations, along the way computing quality measures for every single height value. Unfortunately, this is usually not feasible in practice. Thus, one resorts to the next best solution, namely to fuse DEMs from different providers into a higher-quality product, and estimate its quality in the process.
DEM fusion – and its necessary prerequisite, fine-grained quality characterization of the inputs – has several benefits: improved accuracy, homogeneity and completeness, as well as fine-grained quality information for the final product. We deal only with 2-D surfaces in regular grid format, which constitute the vast majority of large-scale DEMs (although our framework could in principle be extended to TINs).
In this work we make two contributions: First, we develop a computationally efficient and flexible mathematical method for robust fusion of 2.5-D surface models. The formulation is generic and can be applied with any two input DEMs, independent of the sensor technology and processing with which they were created, making it useful for practical applications; it takes into account both prior information about plausible terrain shapes (in the form of a dictionary), and the local accuracy of the inputs, controlled by interpretable weights; and it poses the complete fusion as a clean, convex mathematical optimisation problem that can be solved to global optimality, and in which the influence of the input DEMs is controlled by an interpretable set of local fusion weights. Comparison of the proposed method is done with the data fitting and weighted average methods.
Second, we propose a data-driven method, which allows one to derive local measures of DEM quality (and thus also fusion weights) for each point or segment of a DEM, if no such information is available. To this end we use as input geomorphological characteristics of the terrain (slope, aspect, roughness) which are derived directly from the DEMs, as well as optionally semantic information such as land-cover maps. Using existing high-quality ground-truth DEMs as reference, we learn regression functions relating the available geomorphological characteristics to the DEM quality, which then allow one to estimate the local quality of a new DEM.
The proposed method is evaluated in detail with different datasets, and shows improvement in DEM quality, consistently over all the combinations of inputs.
In an ideal world, one would of course obtain the raw measurements and sensor models from all sensors, and merge them by fitting a single DEM to the entire set of heterogeneous observations, along the way computing quality measures for every single height value. Unfortunately, this is usually not feasible in practice. Thus, one resorts to the next best solution, namely to fuse DEMs from different providers into a higher-quality product, and estimate its quality in the process.
DEM fusion – and its necessary prerequisite, fine-grained quality characterization of the inputs – has several benefits: improved accuracy, homogeneity and completeness, as well as fine-grained quality information for the final product. We deal only with 2-D surfaces in regular grid format, which constitute the vast majority of large-scale DEMs (although our framework could in principle be extended to TINs).
In this work we make two contributions: First, we develop a computationally efficient and flexible mathematical method for robust fusion of 2.5-D surface models. The formulation is generic and can be applied with any two input DEMs, independent of the sensor technology and processing with which they were created, making it useful for practical applications; it takes into account both prior information about plausible terrain shapes (in the form of a dictionary), and the local accuracy of the inputs, controlled by interpretable weights; and it poses the complete fusion as a clean, convex mathematical optimisation problem that can be solved to global optimality, and in which the influence of the input DEMs is controlled by an interpretable set of local fusion weights. Comparison of the proposed method is done with the data fitting and weighted average methods.
Second, we propose a data-driven method, which allows one to derive local measures of DEM quality (and thus also fusion weights) for each point or segment of a DEM, if no such information is available. To this end we use as input geomorphological characteristics of the terrain (slope, aspect, roughness) which are derived directly from the DEMs, as well as optionally semantic information such as land-cover maps. Using existing high-quality ground-truth DEMs as reference, we learn regression functions relating the available geomorphological characteristics to the DEM quality, which then allow one to estimate the local quality of a new DEM.
The proposed method is evaluated in detail with different datasets, and shows improvement in DEM quality, consistently over all the combinations of inputs.