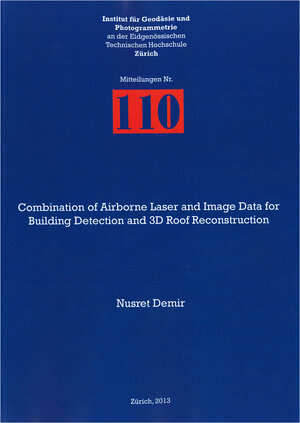
×
Combination of Airborne Laser and Image Data for Building Detection and 3D Roof Reconstruction
von Nusret DemirIn this work, an automated approach for building detection and 3D roof reconstruction using airborne image and laser scanning (also known as LIDAR) data is presented. The approach consists of two main parts:
Building detection is tested using two datasets (Vaihingen and Zurich), whereby the first one included images of a digital sensor, while the second one scanned film images. Both sites included LIDAR data. Additional data were derived, like DSM from image matching, DTM (when not available) from DSM filtering and orthoimages. The reconstruction is tested with one dataset (Vaihingen) using different processing parameters. Zurich was not used for reconstruction, as it has a low density in the LIDAR data (1 point per 2 square meters). In both datasets, reference data were manually collected from the images for quantitative evaluation.
Four different variants of building detection are presented. Each variant has a different workflow and is capable of detecting buildings.
The first variant of building detection is based on multispectral classification and DSM filtering. Here, all image channels are used together with derived channels such as NDVI, the first PC and saturation, in a supervised maximum likelihood classification of the ERDAS software package, to detect buildings. The classification results are improved, especially regarding mixture of buildings with roads and bare ground, using DSM blobs, derived from subtraction of the DTM from the DSM. Small wrong buildings are eliminated using size criteria.
In the second variant, DSM blobs, mainly consisting of buildings and trees, are detected by subtraction of the DTM from the DSM. Then, trees are eliminated using NDVI data, derived from unsupervised ISODATA classification of the multispectral images, while small non-building objects are rejected based on size criteria.
The third variant uses the planimetric density of raw LIDAR DTM data to detect the above-ground objects. In the raw DTM, buildings have no data (voids), while trees have a lower density than other objects. NDVI data is used to distinguish buildings from trees, while smaller objects like cars are eliminated using size criteria.
The fourth variant is like the third one, but uses the vertical density of the raw LIDAR data (all points) to distinguish trees and buildings.
To improve the results, a combination of the four variants using set intersections and unions is performed. The combination was empirical, with consideration of the datasets used in each variant and the advantages and disadvantages of each variant.
In the evaluation, the combination of the four individual results yields 94% and 96% correct detections and an omission error of 12% and 14% for the Zurich and Vaihingen datasets respectively. The results are similar, although the input data differed quite a lot, and the average result quality is quite high. In Vaihingen, the detected building area is enlarged before 3D roof reconstruction, in order to increase the probability that the whole area of each building is detected.
The roofs are assumed to consist of planar parts. Thus, reconstruction is conducted by plane detection with RANSAC, followed by geometric refinement and merging of neighboring segments to reduce over-segmentation. Walls and planes belonging to the ground are then detected and excluded and the roof part outlines are vectorised with the alpha-shapes algorithm. The resulting polygons are refined using 3D straight edges reconstructed by automatic edge extraction and matching, as well as virtual 3D edges from the intersection of adjacent planes. Additionally, 3D corner points constructed by intersection of the 3D lines are used. The 3D edges and corner points are integrated with the plane DSM data and the roof outlines are again detected using the alpha-shapes algorithm. Finally, a regularisation of the roof outlines is performed, although the topological correctness of the roof planes is not enforced.
The quantitative evaluation uses several metrics for both correctness and completeness of the roof polygons and for their geometric accuracy. The average mean and median value for correctness of the roof polygons are 84% and 96% and for completeness 76% and 88%. Apart from quantitative measures, also different cases and problems are analysed and visually presented. The results cannot be easily compared to previous research, however, they are quite satisfactory. A critical factor is the quality of the input data, especially their spatial resolution and accuracy.
The work closes with a summary, advantages and disadvantages of the proposed methods, and a future outlook and open research problems.
Building detection is tested using two datasets (Vaihingen and Zurich), whereby the first one included images of a digital sensor, while the second one scanned film images. Both sites included LIDAR data. Additional data were derived, like DSM from image matching, DTM (when not available) from DSM filtering and orthoimages. The reconstruction is tested with one dataset (Vaihingen) using different processing parameters. Zurich was not used for reconstruction, as it has a low density in the LIDAR data (1 point per 2 square meters). In both datasets, reference data were manually collected from the images for quantitative evaluation.
Four different variants of building detection are presented. Each variant has a different workflow and is capable of detecting buildings.
The first variant of building detection is based on multispectral classification and DSM filtering. Here, all image channels are used together with derived channels such as NDVI, the first PC and saturation, in a supervised maximum likelihood classification of the ERDAS software package, to detect buildings. The classification results are improved, especially regarding mixture of buildings with roads and bare ground, using DSM blobs, derived from subtraction of the DTM from the DSM. Small wrong buildings are eliminated using size criteria.
In the second variant, DSM blobs, mainly consisting of buildings and trees, are detected by subtraction of the DTM from the DSM. Then, trees are eliminated using NDVI data, derived from unsupervised ISODATA classification of the multispectral images, while small non-building objects are rejected based on size criteria.
The third variant uses the planimetric density of raw LIDAR DTM data to detect the above-ground objects. In the raw DTM, buildings have no data (voids), while trees have a lower density than other objects. NDVI data is used to distinguish buildings from trees, while smaller objects like cars are eliminated using size criteria.
The fourth variant is like the third one, but uses the vertical density of the raw LIDAR data (all points) to distinguish trees and buildings.
To improve the results, a combination of the four variants using set intersections and unions is performed. The combination was empirical, with consideration of the datasets used in each variant and the advantages and disadvantages of each variant.
In the evaluation, the combination of the four individual results yields 94% and 96% correct detections and an omission error of 12% and 14% for the Zurich and Vaihingen datasets respectively. The results are similar, although the input data differed quite a lot, and the average result quality is quite high. In Vaihingen, the detected building area is enlarged before 3D roof reconstruction, in order to increase the probability that the whole area of each building is detected.
The roofs are assumed to consist of planar parts. Thus, reconstruction is conducted by plane detection with RANSAC, followed by geometric refinement and merging of neighboring segments to reduce over-segmentation. Walls and planes belonging to the ground are then detected and excluded and the roof part outlines are vectorised with the alpha-shapes algorithm. The resulting polygons are refined using 3D straight edges reconstructed by automatic edge extraction and matching, as well as virtual 3D edges from the intersection of adjacent planes. Additionally, 3D corner points constructed by intersection of the 3D lines are used. The 3D edges and corner points are integrated with the plane DSM data and the roof outlines are again detected using the alpha-shapes algorithm. Finally, a regularisation of the roof outlines is performed, although the topological correctness of the roof planes is not enforced.
The quantitative evaluation uses several metrics for both correctness and completeness of the roof polygons and for their geometric accuracy. The average mean and median value for correctness of the roof polygons are 84% and 96% and for completeness 76% and 88%. Apart from quantitative measures, also different cases and problems are analysed and visually presented. The results cannot be easily compared to previous research, however, they are quite satisfactory. A critical factor is the quality of the input data, especially their spatial resolution and accuracy.
The work closes with a summary, advantages and disadvantages of the proposed methods, and a future outlook and open research problems.